The clinical trial ecosystem faces tremendous pressure and challenges. While scientific advancements continue at an unprecedented pace,1 the operational framework supporting clinical trials remains woefully inefficient. Sites are stretched to their limits, pharmaceutical companies are investing into trials that take too long to complete, and contract research organizations (CROs) are unable to meaningfully accelerate the process. Due to this systemic inefficiency, companies are missing opportunities to deliver more drugs to patients faster, including novel orphan drugs where the traditional economics of pharmaceutical drug development are often prohibitive.2
At the heart of this challenge is the historical reliance on outdated, time-consuming, and error-prone manual processes that hinder scalability. Even as the number of clinical sites, investigators, and research coordinators available to conduct trials is finite, the overall number of clinical studies has grown substantially in the past 2 decades.3 Without a fundamental shift in how trials are managed, these types of bottlenecks will persist or even become worse. Enter artificial intelligence (AI), a technological advancement that we believe offers a way to break past the traditional constraints.
By automating administrative tasks and optimizing workflows, AI technology can significantly increase efficiency.4 This means that research sites can potentially conduct a greater number of trials without needing to scale up staff, while simultaneously improving the quality, reliability, and consistency of data. This is already happening in practice, as some research sites report a 90% reduction in queries per visit and a decrease of query close time from 2 weeks to 2 days.5 This has the potential for faster drug development timelines, more opportunities for groundbreaking therapies, and a transformation in how clinical research is conducted.
Just as electronic data capture systems became a necessity in clinical trials, AI “teammates” are poised to become the next essential tool in modern research. Given the rapid advancements in AI reasoning capabilities,6 a divide will likely form between traditional and AI-powered trial sites. Those sites and investigators that embrace AI teammates will be at the forefront of the future of clinical trials. Conversely, those who fail to adopt this technology risk being left behind in an industry that is quickly evolving.
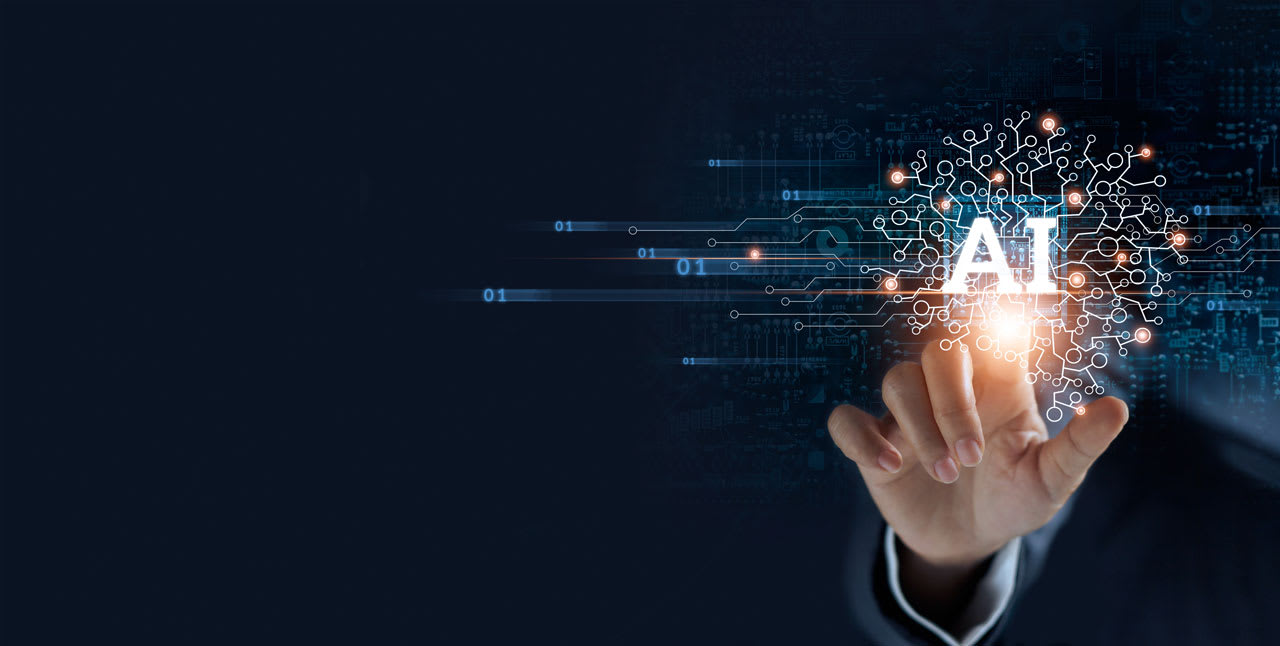
The Growing Divide
Despite its early stage of adoption, AI is already demonstrating measurable benefits in clinical trials.5 Sponsors and research teams implementing AI teammates are accelerating key processes, reducing administrative burden, and improving compliance. For example, AI can optimize site selection, streamline patient recruitment, and automate compliance tracking. Notably, recent research indicates that AI can dramatically accelerate study plan development, reducing the timeline from weeks or months to just a few days.7
This transformation is akin to the automation revolution in manufacturing: once the advantages of efficiency and accuracy became apparent, there was no turning back. The same is happening in clinical trials today. Sites using AI will gain a compounding advantage, as it continuously refines processes and improves over time. Meanwhile, sites staying with traditional, manual methods risk being left behind. The difference between AI-driven and manual research sites will become increasingly stark in the coming years, with sites powered by intelligent automation able to process increasingly higher trial volumes while simultaneously maintaining superior data quality.
Even now, there are early signs of enhanced collaboration between sites using AI teammates and sponsors, with predictive modeling enabling more precise study feasibility assessments and reducing time spent on site activation.8,9 This leads to real-time visibility into each site’s performance, facilitating data-driven decision-making and improving communication. This successful collaboration highlights how the integration of AI in research management has the potential to create a virtuous cycle of enhanced efficiency, higher quality, and improved study outcomes.
AI’s role as an efficiency multiplier in clinical trials has been noted previously, helping to augment site staff and reduce repetitive administrative work. Early site adopters of AI teammates in ophthalmology research report that staff can focus more on core responsibilities such as patient care, protocol adherence, and data integrity.5 They furthermore indicate that removal of repetitive administrative tasks leads to less stress and greater satisfaction for site staff. These results are realized because of AI’s potential to fundamentally redefine clinical trial operations by streamlining 4 key areas: data management, regulatory and compliance work, quality assurance, and staff productivity.
Data Management
Clinical trials generate vast amounts of data, all of which must be meticulously documented and verified. Research sites are often burdened with managing and documenting these data while also responding to and resolving queries. Staff typically work on multiple studies at a time, using various systems to track source data entry, including informed consent forms (ICFs), regulatory documents, startup documents, financial reports, and more. AI teammates are adept at managing data movement from source to electronic data capture (EDC).10 By intelligently automating these processes, they reduce the potential for human error and simultaneously improve data integrity.
By removing the burden of manual data entry and validation from site staff, AI dramatically accelerates the time required to process and analyze data, leading to faster insights and more reliable trial outcomes. It also enables real-time data monitoring, allowing for proactive management of trial progress.
In addition, AI’s natural language processing tools can interpret unstructured data from physician notes and imaging reports. And by analyzing historical patient records it also can increase the number of eligible trial participants. This not only reduces data handling errors but also improves trial design by ensuring that inclusion and exclusion criteria are strictly met.
Regulatory and Compliance Work
Regulatory oversight is a tedious and time-consuming aspect of clinical trials. It also has the potential to cause deviations if consent is done on wrong or outdated forms or if the site does not have appropriate documentation for things such as tracking staff training, delegation of authority, or financial disclosures. AI simplifies compliance by automating documentation, tracking regulatory submissions, and ensuring adherence to protocols. For example, AI teammates can generate and maintain essential trial forms, such as FDA 1572s, delegation logs, and screening logs, automatically flagging missing information or upcoming expirations.
Additionally, AI can provide real-time monitoring of regulatory and compliance requirements, ensuring that trials remain compliant with evolving guidelines. For instance, a major benefit of AI for compliance is with ICF management, historically a challenging issue for studies. AI teammates can interact with Investigational Review Board (IRB) websites and make appropriate workflow decisions when new ICFs are available. This makes certain that patient consents are always in compliance and relieves additional administrative burden from coordinators. AI-driven audits further ensure that trial records remain inspection-ready, minimizing the risk of noncompliance.
Beyond document automation, intelligent compliance monitoring systems can analyze real-time trial execution data to identify potential deviations before they become major issues. This proactive approach reduces protocol violations and improves patient safety, ensuring studies remain on track and mitigating the risk of costly delays or regulatory penalties.
Quality Assurance
Maintaining high-quality data is critical in clinical trials, yet manual processes are prone to errors. AI teammates act as a safeguard, continuously analyzing trial data for inconsistencies, protocol deviations, or missing entries. Predictive analytics enable sites to anticipate potential issues, such as patient dropouts or protocol violations, and take corrective action before problems arise.
This proactive approach to quality management reduces the need for rework and enhances trial efficiency, ensuring that data integrity is preserved throughout the study. AI can also generate real-time quality reports, providing sponsors with transparent insights into trial performance.
Additionally, AI can identify trends in past trials, helping sponsors and CROs refine protocols to reduce complexity and patient burden while maintaining scientific rigor. By leveraging historical data, AI can even suggest modifications that improve patient retention and adherence, reducing dropout rates and enhancing study validity.
Staff Productivity
One of the most significant benefits of AI teammates in clinical research is to support and augment site staff, who often struggle with overwhelming administrative workloads that leave them with reduced time for patient care and study oversight. The technology alleviates this burden by automating routine tasks such as scheduling, patient follow-ups, and document management.
For instance, AI-driven automation can manage patient communications, coordinate trial logistics, and streamline visit scheduling. This results in significant time savings and a more efficient research operation.
AI can additionally help lessen the training burden that new site staff experience through guided, real-time learning paths. For sites that often struggle with employee turnover, training new staff members is a frequent pain point. New staff often require months of training and close oversight, creating an additional burden on existing staff and principal investigators. AI teammates can assist new site staff by streamlining the study processes they have to learn while maintaining adherence to protocols and guidelines. AI-powered support provides contextual guidance, recommending accurate terminology and helping to navigate regulatory documentation.
Furthermore, AI teammates can support site coordinators by providing instant access to protocol details, study requirements, and regulatory guidelines, reducing cognitive overload and minimizing errors. This allows research teams to operate more efficiently, improving both job satisfaction and retention rates.
Conclusion
Research sites that embrace intelligent automation will lead the industry in efficiency, scalability, and data quality. Just as electronic data capture systems became essential in clinical research, AI teammates will soon become a necessity for faster, more reliable clinical trials that bring life-changing therapies to patients sooner. Although AI will not replace human expertise, it will certainly enhance it, allowing researchers to focus on what truly matters: advancing clinical research and bringing new medicines to patients.
As AI continues to evolve, the possibilities for clinical research will expand further. From streamlining patient engagement to optimizing multisite coordination, AI offers a future where research is not constrained by historical processes but instead driven by innovation, efficiency, and quality. RP
References
1. R Street Institute. How AI can advance medical knowledge and improve the patient experience. Accessed April 8, 2025. https://www.rstreet.org/commentary/ai-and-public-health-series-how-ai-can-advance-medical-knowledge-and-improve-the-patient-experience/
2. Althobaiti H, Seoane-Vazquez E, Brown LM, Fleming ML, Rodriguez-Monguio R. Disentangling the cost of orphan drugs marketed in the United States. Healthcare (Basel). 2023;11(4):558. doi:10.3390/healthcare11040558
3. World Health Organization. Number of clinical trials by year, country, WHO region, and income group. Accessed April 8, 2025. https://www.who.int/observatories/global-observatory-on-health-research-and-development/monitoring/number-of-clinical-trials-by-year-country-who-region-and-income-group
4. Chopra H, Annu, Shin DK, et al. Revolutionizing clinical trials: the role of AI in accelerating medical breakthroughs. Int J Surg. 2023;109(12):4211-4220. doi:10.1097/JS9.0000000000000705
5. Yalamanchili R. AI teammates: the future of workflow automation in clinical research with Tilda Research. Presented at: Glaucoma 360 New Horizons Forum; February 7, 2025; San Francisco, CA.
6. Epoch AI. AI benchmarking dashboard. Accessed April 8, 2025. https://epoch.ai/data/ai-benchmarking-dashboard
7. Tilda Research. Internal observations on time savings gained through the use of AI to develop study plans from protocol. Unpublished internal data.
8. Caudill T. Human-enabled AI driving enhancements in clinical trial site selection. The Clinical Trial Vanguard. July 8, 2024. Accessed April 8, 2025. https://www.clinicaltrialvanguard.com/article/human-enabled-ai-driving-enhancements-in-clinical-trial-site-selection
9. Wagner B. AI and ML are transforming clinical research practice. Applied Clinical Trials. May 17, 2023. Accessed April 8, 2025. https://www.appliedclinicaltrialsonline.com/view/ai-and-ml-are-transforming-clinical-research-practice
10. Samman M, Yalamanchili R, Barakat M. Bringing ophthalmic clinical trials into the 21st century: a short explainer. Manuscript submitted for publication.